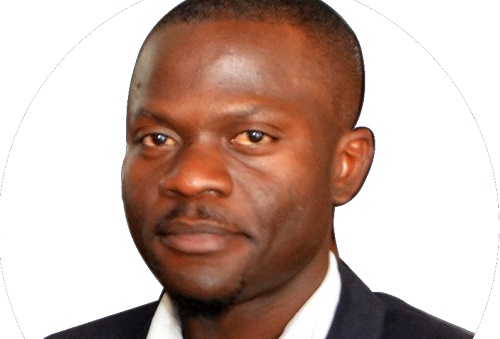
Title: Blablabla Virtualisation
Date and Place: 22/02/2019 at 11:00 in TD-C
Host: Jean-Marie Gorce and Florent de Dinechin
Abstract:
Wired team presentation and discussions about blockchain
La blockchaines sont des technologies de stockage et de transmission d’informations, permettant la constitution de registres répliqués et distribués, sans organe central de contrôle, sécurisées grâce à la cryptographie, et structurées par des blocs liés les uns aux autres, à intervalles de temps réguliers. Elles sont utilisées par un certain nombre d’acteurs et suscitent de très nombreux débats aussi bien au coin café qu’à l’organisation mondiale du commerce.
En tant que membre du laboratoire de recherche CITI, je me sens concerné par ces technologies, et me demande de ce que nous devons en faire.
Je propose dans ce séminaire de vous présenter ma compréhension des systèmes blockchaines et de vous partager mon point de vue de concepteur d’applications distribuées et pair-à-pair sur le Web.
Stéphane Frénot a participé à la création du CITI en 2001. Il est spécialisé dans le génie logiciel et les application distribuées. Il a été responsable du thème middleware et de l’équipe INRIA Amazones au laboratoire. Puis il a participé au projet exploratoire INRIA Dice sur les plateformes d’intermédiations. Depuis 1an il est directeur du département Télécommunications Service et Usages de l’INSA où il enseigne le génie logiciel, les systèmes distribués et l’innovation.
Il a travaillé sur les architectures à composants logiciels, les systèmes pairs-à-pairs pour le déploiement de composants et sur un modèle de programmation orienté flux pour Javascript. Il a participé au dépôt de trois brevets : dans l’iot domestique, dans les flux javascript et dans un protocole de vote. Enfin il est responsable du développement de la plateforme Jumplyn de gestion de projets étudiants actuellement en test sur l’INSA pour la gestion des stages.
Wireless Networks Design in the Era of Deep Learning: Model-Based, AI-Based, or Both?
This work addresses the use of emerging data-driven techniques based on deep learning and artificial neural networks in future wireless communication networks. In particular, a key point that will be made and supported throughout the work is that data-driven approaches should not replace traditional design techniques based on mathematical models. On the contrary, despite being seemingly mutually exclusive, there is much to be gained by merging data-driven and model-based approaches. To begin with, a detailed presentation is given for the reasons why deep learning based on artificial neural networks will be an indispensable tool for the design and operation of future wireless communications networks, as well as a description of the recent technological advances that make deep learning practically viable for wireless applications. Our vision of how artificial neural networks should be integrated into the architecture of future wireless communication networks is presented, explaining the main areas where deep learning provides a decisive advantage over traditional approaches. Afterwards, a thorough description of deep learning methodologies is provided, starting with presenting the general machine learning paradigm, followed by a more in-depth discussion about deep learning. Artificial neural networks are introduced as the peculiar feature that makes deep learning different and more performing than other machine learning techniques. The most widely-used artificial neural network architectures and their training methods will be analyzed in detail. Moreover, bridges will be drawn between deep learning and other major learning frameworks such as reinforcement learning and transfer learning. After introducing the deep learning framework, its application
to wireless communication is addressed. This part of the work first provides the state-of-the-art of deep learning for wireless communication networks, and then moves on to address several novel case-studies wherein the use of deep learning proves extremely useful for network design. In particular, the connection between deep learning and model-based approaches is emphasized, proposing several novel techniques for cross-fertilization between these two paradigms. For each case-study, it will be shown how the use of (even approximate) mathematical models can significantly reduce the amount of live data that needs to be acquired/measured to implement data-driven approaches. For each application, the merits of the proposed approaches will be demonstrated by a numerical analysis in which the implementation and training of the artificial neural network used to solve the problem is discussed. Finally, concluding remarks describe those that in our opinion are the major directions for future research in this field.