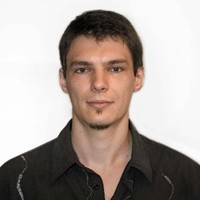
The defense will take place in amphitheater Chappe and you are all welcome to attend as long as there are enough place (35 persons max).
It will be also streamed live on youtube at: https://youtu.be/fvCz5ZXYN_I
Title
Deep Multi-Agent Reinforcement Learning for Dynamic and Stochastic Vehicle Routing Problems
Abstract
Routing delivery vehicles in dynamic and uncertain environments like dense city centers is a challenging task, which requires robustness and flexibility. Such logistic problems are usually formalized as Dynamic and Stochastic Vehicle Routing Problems (DS-VRPs) with a variety of additional operational constraints, such as Capacitated vehicles or Time Windows (DS-CVRPTWs). Main heuristic approaches to dynamic and stochastic problems simply consist in restarting the optimization process on a frozen (static and deterministic) version of the problem given the new information. Instead, Reinforcement Learning (RL) offers models such as Markov Decision Processes (MDPs) which naturally describe the evolution of stochastic and dynamic systems. Their application to more complex problems has been facilitated by recent progresses in Deep Neural Networks, which can learn to represent a large class of functions in high dimensional spaces to approximate solutions with high performances. Finding a compact and sufficiently expressive state representation is the key challenge in applying RL to VRPs. Recent work exploring this novel approach demonstrated the capabilities of Attention Mechanisms to represent sets of customers and learn policies generalizing to different configurations of customers. However, all existing work using DNNs reframe the VRP as a single-vehicle problem and cannot provide online decision rules for a fleet of vehicles.
In this thesis, we study how to apply Deep RL methods to rich DS-VRPs as multi-agent systems. We first explore the class of policy-based approaches in Multi-Agent RL and Actor-Critic methods for Decentralized, Partially Observable MDPs in the Centralized Training for Decentralized Control (CTDC) paradigm. To address DS-VRPs, we then introduce a new sequential multi-agent model we call sMMDP. This fully observable model is designed to capture the fact that consequences of decisions can be predicted in isolation. Afterwards, we use it to model a rich DS-VRP and propose a new modular policy network to represent the state of the customers and the vehicles in this new model, called MARDAM. It provides online decision rules adapted to the information contained in the state and takes advantage of the structural properties of the model. Finally, we develop a set of artificial benchmarks to evaluate the flexibility, the robustness and the generalization capabilities of MARDAM. We report promising results in the dynamic and stochastic case, which demonstrate the capacity of MARDAM to address varying scenarios with no re-optimization, adapting to new customers and unexpected delays caused by stochastic travel times. We also implement an additional benchmark based on micro-traffic simulation to better capture the dynamics of a real city and its road infrastructures. We report preliminary results as a proof of concept that MARDAM can learn to represent different scenarios, handle varying traffic conditions, and customers configurations.
Jury
- François Charpillet, Research Director at INRIA Nancy Grand Est, Reviewer
- Romain Billot, Professor at IMT Atlantique, Reviewer
- René Mandiau, Professor at Université Polytechnique des Hauts de France, Examiner
- Aurélie Beynier, Associate Professor at Sorbonne Université, Examiner
- Christian Wolf, Associate Professor at INSA de Lyon, Examiner
- Olivier Simonin, Professeur à l’INSA de Lyon, Thesis director
- Jilles Dibangoye, Associate Professor at INSA de Lyon, Co-supervisor
- Laëtitia Matignon, Associate Professor at Université Lyon 1, Co-supervisor
- Florian Pereyron, Research Engineer at Volvo Group, Co-supervisor