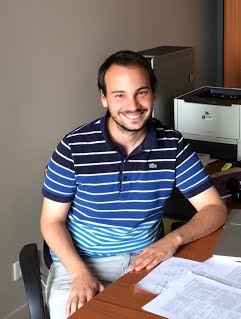
Title: Self trigger co-design using LASSO regression
Date and Place: 17th December 2020 14:00 – link
Speaker: Lucien Etienne (IMT Lille-Douai)
Abstract:
Networked systems have become more and more pervasive in many modern industrial application. A good justification for their deployment is that they can be cheaper/faster to set in place as well are scalable while also enabling lower maintenance cost. In the past decade a new paradigm has been developed where the controller is not sampled periodically (i.e. with a time–triggered policy), but rather sampled when some condition has been met (Usually a stability or performance criterion being violated). After recalling some general element on classical control scheme ( Linear Quadratic regulator and model predictive control) In this talk, the control of a linear time invariant system with self triggered sampling is considered .
In order to address the controller computation and the future sampling schedule a sparse optimization problem will be considered. A relaxation of the optimal self triggered control can be formulated as a LASSO regression. Using the properties of the solution of the Lasso regression it is shown how to obtain a controller ensuring practical or asymptotic stability while reducing sampling of the control action.
Biography:
Dr. Lucien Etienne received a M.Sc. Degree in applied mathematics at the INSA Rouen in 2012 and a joint Ph.D. in automatic control from the university of L’aquila and the university of Cergy-Pontoise in 2016. From 2016 to 2017 he was a post doctoral researcher at Inria Lille-Nord Europe. Since 2017 He is an associate professor at Institut Mines-Télécom Lille Douai. His research interests include switched and hybrid systems, observer synthesis and sampled data systems.