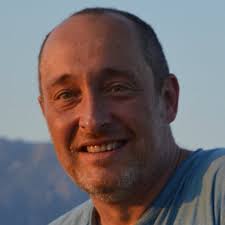
Title: On computing individual exposure risk to a pandemia with BLE-RSSI measures
Date and Place: 07 / 07 / 2020 14:00
Speaker: Jean Marie GORCE (Citi)
Place: https://bbb.tuxlab.net/b/mal-eef-nrd
Abstract: Tracking how Covid-19 spreads over a population is a critical aspect that may aid relaxing lockdown conditions. Most of current solutions (e.g. as proposed in the European project PEPP-PT, the French application Stopcovid, the GAEN (Google-Apple Exposure Notification) ) rely on the RSSI signals obtained with Bluetooth Low Energy (BLE) HELLO messages. In this talk, we describe an algorithm which is complient with the constraints imposed by the rules and parameters of the ROBERT protocol developed by the Privatics team (Inria) and used in the Stopcovid application. Underlying the algorithm is mathematical modeling of the physical wireless communication link, based on real-life BLE RSSI traces. In particular, the algorithm is evaluated on experimental data obtained in the PEPP-PT project (April 2020) and lead by the Fraunhoffer institute, and experimental data aquired by the Stopcovid consortium (May, 2020) lead by Inria, both providing a large number of device-to-device BLE RSSI traces in realistic scenarios.