Title Clustering and Data Anonymization by Mutual Information Abstract In this talk, we first introduce the Shannon theoretic multi-clustering problem and investigate its properties, uncovering connections with many other coding problems in the literature. The figure of merit for this information-theoretic problem is mutual information, the mathematical properties of which make the multi-clustering problem amenable to techniques that could not be used in a general rate-distortion setting. We start by considering the case of two sources, where we derive singleletter bounds for the achievable region by connecting our setting to hypothesis testing and pattern recognition recognition problems in the information theory literature. We then generalize the problem setup to an arbitrary number of sources and study a CEO problem with logarithmic loss distortion and multiple description coding. Drawing from the theory of submodular functions, we prove a tight inner and outer bound for the resulting achievable region under a suitable conditional independence assumption. Furthermore, we present a proof of the well-known two-function case of a conjecture by Kumar and Courtade (2013), showing that the dictator functions are essentially the only Boolean functions maximizing mutual information. The key step in our proof is a careful analysis of the Fourier spectrum of the two Boolean functions. Finally, we study information-theoretic applications to the problem of statistical data anonymization via mutual information and deep learning methods in which the identity of the data writer must remain private even from the learner. Joint works with Dr. Georg Pichler (TU Wien, Austria), Prof. Gerald Matz (TU Wien, Austria), Clément Feutry (CentraleSupélec, France) and Yoshua Bengio (Montréal, Canada) Short biography Pablo Piantanida received both B.Sc. in Electrical Engineering and B.Sc. in Mathematics degrees from the University of Buenos Aires (Argentina) in 2003, and the Ph.D. from Université Paris-Sud (Orsay, France) in 2007. Since October 2007 he has joined the Laboratoire des Signaux et Systèmes (L2S), at CentraleSupélec together with CNRS (UMR 8506) and Université Paris-Sud, as an Associate Professor of Network Information Theory. He is an IEEE Senior Member, coordinator of the Information Theory and its Applications group (ITA) at L2S, and coordinator of the International Associate Laboratory (LIA) of the CNRS “Information, Learning and Control” with several institutions in Montréal and General Co-Chair of the 2019 IEEE International Symposium on Information Theory (ISIT). His research interests lie broadly in information theory and its interactions with other fields, including multi-terminal information and Shannon theory, machine learning, statistical inference, communication mechanisms for security and privacy, and representation learning.
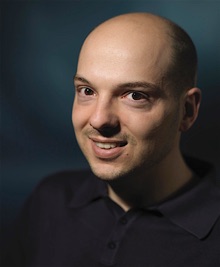